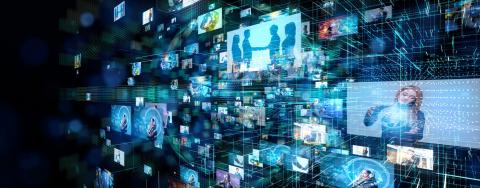
Computer Vision: A Growing Field of Research
Interview with Matthieu Cord.

With the advancement of artificial intelligence (AI), machines are now not just capable of recognizing objects and locating them; they can now analyze and interpret images, too.
Matthieu Cord, professor at the Institute of Intelligent Systems and Robotics (ISIR), and researcher at the valeo.ai laboratory, is here to help us better understand how machines are gradually opening their eyes to our world.
What have been some of the milestones in the development of computer vision?
Matthieu Cord : Computer vision began in the 1980s with "geometric image understanding," which did not require any training. The machine had to be able to provide each pixel in an image’s information with the depth of the scene photographed (foreground or background). Then, in the 90s and 2000s, computer vision researchers began wanting to recognize shapes and objects in order to interpret the content of images. And this is where our encounter with statistical learning became decisive. In order to transform a starting image into semantic information (what the image represents, for example), a mathematical function is used, with many coefficients. If you change the values of these coefficients, the result changes. The aim is for the machine to learn to adjust these parameters so that each time it is presented with an image, it correctly identifies its content.
With so-called supervised learning, the machine is trained on millions of "labelled" images, i.e. images to which the expected result has been assigned beforehand. It is guided by indicating for each image whether its answer is correct. At the beginning, the machine systematically makes mistakes. Then it learns from its mistakes, adjusting its parameters to improve its performance. As its training progresses, the number of errors decreases until the machine no longer makes mistakes, and is even able to generalize its learning enough to apply it to new cases. It can then be used to recognize objects, faces and more.
What is computer vision?
At the intersection of mathematics and computer science, computer vision is a branch of artificial intelligence that deals with image processing. Its objective is to extract, from raw data (digital images or videos), relevant information that can be interpreted and used by a computer or a robot.
What are the latest developments in this field?
M. C. : These supervised learning techniques have become extremely powerful and can equal or even surpass human vision. However, they rely on the use of labelled databases that are often gigantic. One of the current fields of research is therefore to make the machine more autonomous in its learning. This is called "unsupervised learning": the data is communicated to the machine without the need to provide it with explicit supervision. Innovative solutions have emerged in this direction. For the moment, they don't work as well as fully supervised learning, but they are very promising.
Can you tell us more about the research and teaching chair you hold?
M. C. : I am a laureate of the National AI 2020 Chair entitled "VISA-DEEP: Towards Visual Reasoning in Deep Learning.” Deep learning is based on a network of artificial neurons, composed of tens or even hundreds of “layers” of neurons (hence the term “deep”), stacked one on top of the other and interdependent. This network is an example of the mathematical function mentioned above with many parameters to be learned (hence the term “learning”). In the chair that I manage, we use this method not only to ensure that the machine recognizes or locates an object, but that it operates more advanced mechanisms, a form of reasoning in relation to objects. For example, if the task is not only to detect the people in an image but to know how many are sitting next to the window, this implies detecting the people, the window, but also what "near" or "sitting", means, for example, and therefore having some form of reasoning.
What are the different applications for computer vision?
M. C. : There are many applications in many fields of society. Computer vision is used, for example, for the development of autonomous mobility (such as cars, trains, shuttles and drones), for robots in industry, or those that are sent to high-risk sites. It is also used in the fields of security and defense, notably through facial recognition. Computer vision is also widely used in the health sector. For example, it can be used to assist doctors with diagnosis or during operations. Within Sorbonne University, SCAI brings together and coordinates many interdisciplinary initiatives in AI. For my part, I have a collaboration with Prof. Lionel Naccache who works at the Brain and Spinal Cord Institute on problems of functional neuroimaging, and my colleague Patrick Gallinari heads a chair on the theme of AI and climate at ISIR.
But computer vision can also be used in playful contexts. For example, with my team, I have developed an application that allows you to obtain the recipe for a dish you’ve taken a photo of with your smartphone.
Computer vision raises ethical questions. How do you take them into account in your research?
M. C. : The AIs we make are shaped by the data we provide to the machine. But the way in which we choose them is not neutral. It can carry biases, some of which pose serious problems when used in an uncontrolled or inappropriate way. This is why we have developed a focus on the detection of biases and how to take them into account. My doctoral candidate Corentin Dancette has recently published a new learning strategy that makes it possible to correct certain biases. The ethical questions that arise in connection with AI go far beyond this framework. My colleague at ISIR, Raja Chatila, is doing remarkable work on a wide range of topics such as robot autonomy, facial recognition and machine energy consumption. All my doctoral candidates are very concerned about the ethical issues that arise in AI, and I think it’s a good thing!